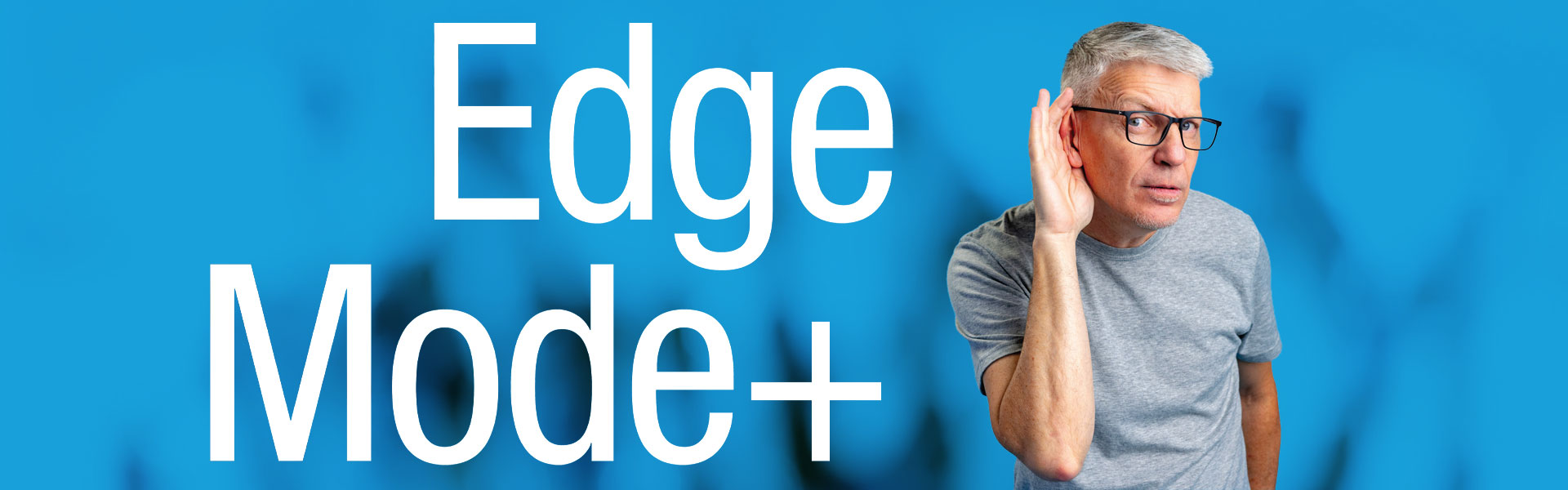
Edge Mode+: On-demand processing improves speech recognition and listening effort in hearing-aid users
Brittany N. Jaekel, M.S. Ph.D., Jingjing Xu, Ph.D.
Introduction
Understanding speech in noise remains difficult for hearing aid users.1 Per the MarkeTrak 2022 survey, hearing aid users were least satisfied with their ability to hear in the following listening situations: in a classroom or movie theater (70% satisfaction), conversations in noise or conversations with large groups (72% satisfaction), and in a lecture hall (72% satisfaction) – that is, environments that may be noisy and/or reverberant and contain many talkers.1 While hearing aids have sophisticated noise reduction strategies, the MarkeTrak 2022 survey also found that the noise reduction capability of hearing aids was an area of least satisfaction among hearing aid users queried about the sound quality of their devices (i.e., 23% of respondents reported being dissatisfied or neutral on this metric).1
Beyond noise reduction strategies, acoustic environmental classification (AEC) is a feature of modern hearing aids that identifies and classifies the current listening environment, and then automatically applies environment-specific changes to the hearing aid’s gain, microphone settings, and compression, in addition to noise reduction settings.2 These environment-specific changes aim to improve speech intelligibility in a variety of listening situations; however, the classifier may sometimes be inaccurate, or the adjustments made by AEC may not be strong enough to combat noise in particularly challenging edge cases,2 resulting in unsatisfactory outcomes.
In order to address these unmet needs, implementing an on-demand option for hearing aid users is highly desirable. One tool aiming to help Starkey hearing aid users in the abovementioned challenges is called Edge Mode+, which is an on-demand processing feature guided by artificial intelligence (AI).
How Edge Mode+ Works
Edge Mode+, when activated by the hearing aid user, prompts the hearing aid to classify the current acoustic environment and then apply additional specialized setting changes to noise reduction, directional microphone, gain, etc., over and above those supplied by AEC. These specialized setting changes are specific to the listener’s current environment as well as the listener’s goal, thus optimizing the user’s listening experience. For example, a listener wanting to hear people more clearly might select Edge Mode+ Enhance Speech, while a listener wanting even more comfort in noise might select Edge Mode+ Reduce Noise. These classification and adaptation schemes were derived via machine learning, in which models were trained on a large number of real-life sound recordings, and further refined via input from both practitioners and listeners.
One goal of Edge Mode+ is to improve user outcomes – like speech understanding and listening effort – in the most difficult listening scenarios, while also adjusting for the user’s intent for the listening interaction. In this article, results from two lab-based studies demonstrate the benefits of the Edge Mode+ feature in Starkey’s Genesis AI hearing aids.
Study 1: Edge Mode+ Improves Speech Recognition
The first study assessed whether Edge Mode+ Enhance Speech improves listeners’ speech recognition in noise.
Twelve experienced hearing aid users (5 females, 7 males) wore Starkey Genesis AI 24 receiver-in-the-canal (RIC) devices (programmed to Starkey’s proprietary fitting formula, e-STAT 2.0) with audiometrically appropriate occluded earbuds or earmolds. In general, participants had sloping mild to moderately severe hearing losses (Figure 1), and their age range was 42 to 84 years, with a mean of 71.1 years and standard deviation of 12.2 years.
For this experiment, the participants’ task was to repeat sentences presented in the context of a real-life recording of restaurant noise. This noise was unique in that it included several noisy distractions that would be typical in a restaurant setting: people talking in the background, radio music playing, and clattering dishes. The target speech was always presented from the loudspeaker directly in front of the seated participant (0°), while noise was presented from the seven loudspeakers surrounding the participant (spaced at 45° intervals).
The restaurant noise was presented at 63 dB-A (summated). The level of the target speech was individualized for each participant. Specifically, speech was presented at the level needed for that participant to achieve approximately 70% correct speech recognition while listening in the restaurant noise with default hearing aid settings. This level was chosen to ensure that the listening task was neither too easy nor too difficult for participants. A brief pre-test was administered to determine each participant’s individualized target speech level.
The target speech was lists of IEEE sentences3 spoken by a female native speaker of American English. After each sentence, participants repeated what they heard, and were scored on the number of keywords reported back correctly (out of 5 keywords per sentence). Participants performed this speech task twice: once with default hearing aid settings (i.e., Edge Mode+ was disabled) and once with Edge Mode+ Enhance Speech enabled. The order of testing was randomized, and participants were masked to condition.
In the default hearing aid settings condition, participants, on average, recognized 62.6% of the speech in restaurant noise (Figure 2). When Edge Mode+ Enhance Speech was enabled, speech recognition improved by 10.7 percentage points to 73.3%. To analyze this change statistically, scores were transformed into rationalized arcsine units (RAUs), which can be interpreted similarly to percentages and allow for parametric statistical testing.4 The improvement in speech understanding with Edge Mode+ Enhance Speech was statistically significant (t(11)=2.55, p=0.027). Thus, enabling Edge Mode+ Enhance Speech allowed participants to understand significantly more speech in a realistic, noisy background.
Study 2: Edge Mode+ Improves Listening Effort
The second study assessed whether Edge Mode+ could improve (i.e., reduce) listening effort in noisy environments. Listening effort has been defined as “the mental exertion required to attend to and understand an auditory message.”5 If Edge Mode+ can process incoming signals such that it is easier for the listener to understand speech, then mental exertion – or listening effort – should be reduced with Edge Mode+ compared to default hearing aid settings.
Twenty experienced hearing aid users (5 females, 15 males) participated in this study, and were fit with the same devices, fitting formula, and coupling strategy as described in Study 1. On average, participants had sloping mild to moderately severe hearing losses (Figure 1), and their age range was 42 to 84 years, with a mean of 71.2 years and standard deviation of 12.7 years. Note that a subset of these participants was also tested in Study 1.
The experimental task was the Adaptive Categorical Listening Effort Scaling (ACALES) test.6 For this test, participants listened to English Matrix Test sentences7 presented in a modulated background noise. While the noise remained fixed at a constant level, the loudness of the sentences varied across a range of signal-to-noise ratios (SNRs). After each SNR presentation, participants rated their listening effort: specifically, they were prompted to answer the question, “How much effort does it require for you to follow the speaker?” using a 13-point scale, ranging from “1 = No effort” to “13 = Extreme effort”. A fourteenth option, “Only noise”, was also available to participants, for situations when the speech was so soft in level that participants could perceive nothing but noise.
Participants completed the ACALES test twice: once with default hearing aid settings (i.e., Edge Mode+ was disabled), and once with Edge Mode+ Enhance Speech enabled. The test order was randomized and participants were masked to condition.
An Example
The type of data collected via the ACALES test is shown in an illustrative example in Figure 3 (that is, the information presented in Figure 3 is for explanatory purposes only and does not reflect actual data collected in the experiment). Listening effort ratings are plotted as a curve, demonstrating how listening effort ratings change across SNRs. In this example, the highest listening effort (ratings 9 to 13, considerable effort to extreme effort) are associated with the most negative (most difficult) SNRs, and the lowest listening effort (ratings 1 to 5, no effort to little effort) are associated with the most positive (most easy) SNRs.
When comparing ACALES outcomes across listening conditions, inspecting how the curve shifts along the SNR axis for each condition allows us to interpret whether listening effort has improved or worsened with each condition. For example, as shown in Figure 3, if the comparative condition’s curve shifts leftward on the SNR axis, then that condition allowed for improved (reduced) listening effort. In other words, the listener’s ratings have changed such that listening to speech in more difficult SNRs has become easier (less effortful).
Experimental Results
For statistical analysis, mean SNR benefits were derived from the ACALES outcomes. For each participant, the mean SNR benefit of Edge Mode+ Enhance Speech, as compared to default hearing aid settings, was calculated as:
where n was the number of listening effort ratings for the fitted curve, and SNR defaulti and SNR Edge Mode+ Enhance Speechi were the SNRs for effort rating score i. A positive mean SNR benefit value indicated the benefit of using Edge Mode+ Enhance Speech over default settings.
Figure 4 shows average mean SNR benefits for Edge Mode+ Enhance Speech over default settings. Overall, compared to default settings, the average mean SNR benefit with Edge Mode+ Enhance Speech was +1.13 dB (t(19) = 2.08, p = 0.05). This indicated that Edge Mode+ Enhance Speech improved (reduced) listening effort, on average. Note that a difference of approximately +1 dB is associated with an approximate 13% increase in speech understanding on the English Matrix Test.8
While we found an overall significant benefit for Edge Mode+ Enhance Speech for listening effort, we observed that individual mean SNR benefits varied across our 20 participants. As an example, in Figure 5, we present results from two participants with similar hearing losses, but who show very different levels of benefit with Edge Mode+ Enhance Speech. Participant #1 experienced an SNR benefit with Edge Mode+ Enhance Speech only in conditions perceived to require the highest listening effort, while Participant #2 experienced SNR benefits with Edge Mode+ Enhance Speech across all levels of listening effort. Thus, as an on-demand feature, Edge Mode+ can likely provide significant benefit for those patients who need additional assistance in noisy situations.
Conclusion
While the MarkeTrak 2022 survey data indicate that more than 80% of hearing aid owners are satisfied with their devices, hearing aid wearers still encounter difficulties understanding speech in certain noisy environments.1 Edge Mode+, as an AI-driven, on-demand feature, provides an option for hearing aid users to optimize their hearing aids in situations where they have trouble hearing with the default settings. More importantly, Edge Mode+ in Genesis AI 24 and 20 tier devices provides choices (Enhance Speech and Reduce Noise) that can take the user’s listening intent into consideration, which can further tailor the signal processing to meet the wearer’s needs.
Results from the present studies showed that compared to the default hearing aid settings, the Edge Mode+ Enhance Speech feature improved speech understanding and listening effort in noisy conditions. These findings support the conclusion that Edge Mode+ can provide additional speech enhancement and noise reduction to help communication in these challenging situations. ■
References
- Picou EM. Hearing Aid Benefit and Satisfaction Results from the MarkeTrak 2022 Survey: Importance of Features and Hearing Care Professionals. Semin Hear. 2022;43(04):301-316. doi:10.1055/s-0042-1758375
- Fabry DA, Bhowmik AK. Improving Speech Understanding and Monitoring Health with Hearing Aids Using Artificial Intelligence and Embedded Sensors. Semin Hear. 2021;42(03):295-308. doi:10.1055/s-0041-1735136
- Rothauser E, Chapman W, Guttman N, Hecker MHL, Nordby KS, Silbiger HR, Urbanek GE, Weinstock M. IEEE Recommended Practice for Speech Quality Measurements. IEEE Transactions on Audio and Electroacoustics. 1969;17(3):225-246. doi:10.1109/tau.1969.1162058
- Studebaker GA. A "rationalized" arcsine transform. J Speech Hear Res. 1985;28(3):455-62. doi:10.1044/jshr.2803.455
- McGarrigle R, Munro KJ, Dawes P, Stewart AJ, Moore DR, Barry JG, Amitay S. Listening effort and fatigue: what exactly are we measuring? A British Society of Audiology Cognition in Hearing Special Interest Group 'white paper'. Int J Audiol. 2014;53(7):433-40. doi:10.31 09/14992027.2014.890296
- Krueger M, Schulte M, Brand T, Holube I. Development of an adaptive scaling method for subjective listening effort. J Acoust Soc Am. 2017;141(6):4680. doi:10.1121/1.4986938
- Kollmeier B, Warzybok A, Hochmuth S, Zokoll MA, Uslar V, Brand T, Wagener KC. The multilingual matrix test: Principles, applications, and comparison across languages: A review. Int J Audiol. 2015;54 Suppl 2:3-16. doi:10.3109/14992027.2015.1020971
Jingjing Xu, Ph.D., is a research scientist in the department of clinical and audiology research at Starkey. Before joining Starkey in 2016, he was a research assistant professor of audiology at the University of Memphis. He received his master's degree in Engineering Acoustics from the Technical University of Denmark and his Ph.D. in Communication Sciences and Disorders from the University of Memphis. His research interests include acoustics, speech recognition, hearing aid outcome measures, and ecological momentary assessment.
Brittany N. Jaekel, M.S. Ph.D., joined Starkey as a research scientist in 2021. She earned her master’s degree in Communication Sciences and Disorders from the University of Wisconsin-Madison and her Ph.D. in Hearing and Speech Sciences from the University of Maryland-College Park. Her research has focused on speech perception outcomes in people with hearing prostheses and the impacts of aging on communication.